Table of Content
TABLE OF CONTENTS
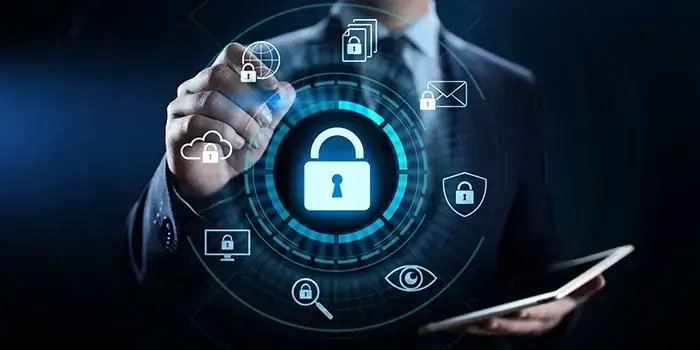
Overview
Financial fraud is expensive, not just in terms of the money involved, but also for poor customer experience and loss of customer trust. There are many types of financial frauds, such as credit card frauds, e-commerce frauds, insurance frauds, to name a few.
Fraud techniques are continually evolving; they may involve an individual or a group of people working together to commit a fraudulent transaction. It is not only essential to detect fraud but to be able to prevent it. It is cardinal to think one step ahead and detect fraud early before significant losses occur.
In this blog, we will be discussing a working example of one of the leading methods of committing fraud, "Fraud Rings." A fraud ring is an organized way of committing forgery, filing false claims, identity stealing, or counterfeiting checks and currencies.
This blog attempts to demonstrate how Knowledge Graphs/Graph theories help in detecting potential fraudsters.
The fundamental structure, also called a graph's topology, gives many insights that are not easily discoverable, just by examining data in tabular formats. Rings in graphs are a powerful means to highlight subgraphs which have nodes with common characteristics. These common characteristics can indicate family members, groups of loyal customers, or incidents of identity fraud. Such rings in subgraphs are known as Fraud Rings and are very useful for uncovering many different types of fraud across various channels.
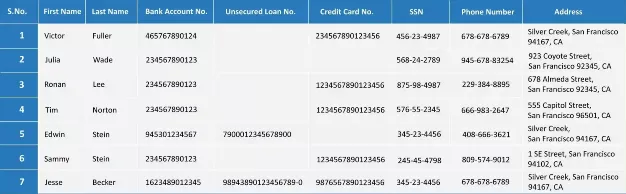
(All the numbers given above are fictional)
The general tendency is that a group of people working collectively to commit a fraud might use overlapping identity documents. This may be because of deliberately generated synthetic identities or stolen identities arranged to support their criminal intentions. Our example here shows synthetic data of three account holders, who have opened separate accounts and have been issued credit cards or unsecured loans by the bank using overlapping supporting/identity characteristics like phone number, SSN, and address.
As seen from the above table, finding shared values for different properties is not very obvious. With millions of rows of data in real financial datasets, the discovery of such patterns would be very complicated. However, Knowledge Graphs can be a potent tool to identify and represent such connections.
We have implemented a simple link analysis to uncover fraud rings. The Knowledge Graph demonstrates the presence of rings in the data.
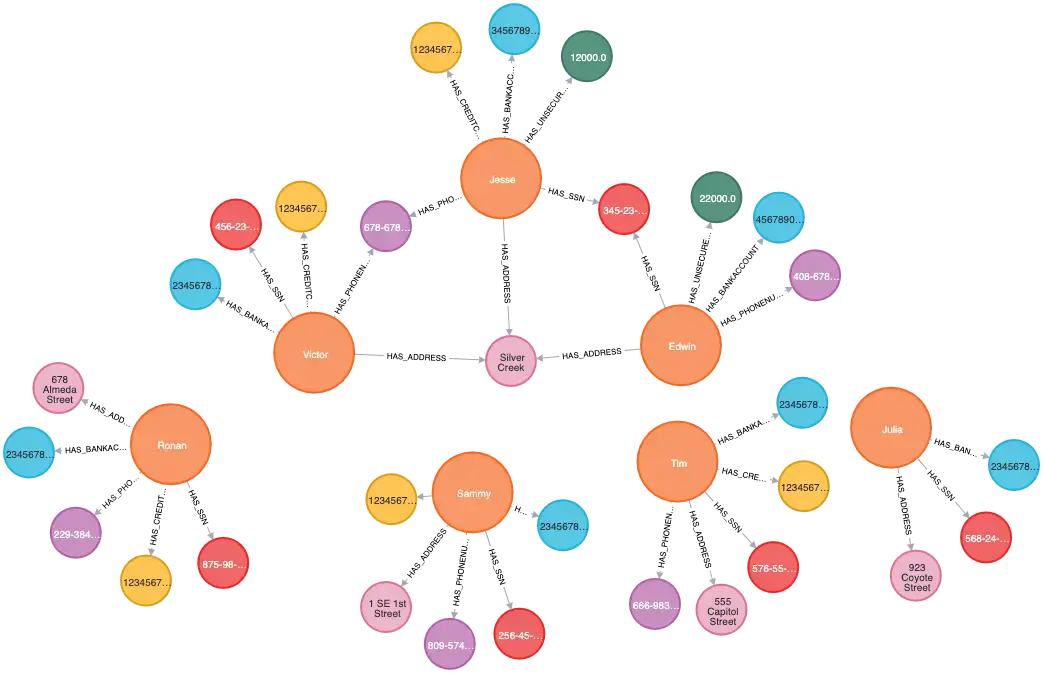
In the above Knowledge Graph, three people share some properties leading to a graph ring. On examining the common properties, it may be inferred that having a common address can indicate that these people belong to the same family. However, a common SSN can be a potential alert for further investigation. Thus, such rings in the Knowledge Graph can quickly provide powerful insights about the data.
In this case, we even calculated the financial risk involved in each of the rings identified by adding the credit card limit and the loan balance amount.
Fraud Ring | Contact Type | Ring Size | Financial Risk |
[Matt Smith, Jane Appleseed, John Doe] | [Address] | 3 | 34387.0 |
[Matt Smith, John Doe, Jane Appleseed] | [Address] | 3 | 34387.0 |
[Matt Smith, Jane Appleseed] | [SSN] | 2 | 29387.0 |
[Matt Smith, Jane Appleseed] | [SSN] | 2 | 29387.0 |
[Jane Appleseed, John Doe] | [PhoneNumber] | 2 | 18046.0 |
[Jane Appleseed, John Doe] | [PhoneNumber] | 2 | 18046.0 |
Conclusion
The advantage of using Knowledge Graphs for detecting fraud rings goes beyond individual data points to connections that link them. It may hence help uncover high financial frauds quickly. Similarly, fraud detection and analysis can be done at different points in time, such as for every new account, or whenever any credit threshold is reached or periodically as a back-end process.
Such Knowledge Graph-based analysis may also support discovering cross channel frauds. There can be many other ways to uncover frauds, and many ML/AI approaches can be combined with graphs to identify and prevent frauds efficiently.
It's all about Architecting Enterprise Intelligence!
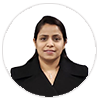
Deepti Soni
Director, Data Science and Analytics
Deepti Soni is a Data Science professional with more than ten years of experience in architecting innovative analytical solutions for strategic business problems.